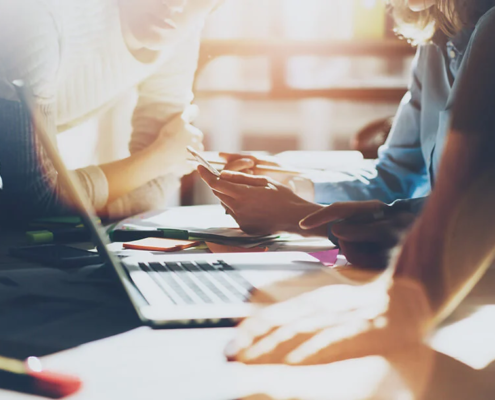
Anything we can do to better learn how people interact with us gives us behavioral intelligence that will drive discovery. This discovery is a powerful way to identify and predict behavior, and helps researchers in all fields. This drive to understand and predict behaviors and its unique ability to accelerate discovery is why Machine Learning is a fundamental strategy for today and tomorrow.
People ask me all the time, can a machine really learn? The answer is an absolute yes! Machines can be programmed to learn by studying data to detect patterns and by applying known rules to:
• Categorize or catalog people or things
• Predict likely outcomes or actions based on identified patterns
• Identify hitherto unknown patterns and relationships
• Detecting anomalous or unexpected behaviors
The processes machines use to learn are known as algorithms and functions. As new observations or changes to the environment are provided to the “machine” the algorithm’s performance improves. Thereby resulting in increasing “intelligence” over time.
With the advent of big data and lower costs of storage and processing, the amount of data available and our ability to process it has increased exponentially. The ability of machines to learn and thus appear ever more intelligent has increased proportionally. Even so, machines aren’t independent thinkers (yet). Yes, machine learning may identify previously unidentified opportunities or problems to be solved. Machines are not autonomously creative and they will not spontaneously develop new hypotheses from facts (data) not in evidence. Nor can the machine determine a new way to respond to emerging stimuli. Remember: the output of a machine learning algorithm is entirely dependent on the data it is exposed to. Change the data, change the result. Machine-learning tools can also show false positives, blind alleys and mistakes since many of the algorithms are so complicated that it is impossible to inspect all the parameters or to determine how the inputs have been manipulated. As these algorithms begin to be applied ever more widely, risks of misinterpretations, erroneous conclusions and wasted scientific effort will spiral.
Companies are better than ever at understanding why customers buy their products, use their services, or engage their expertise. We can point the “machine” at a lake of consumer data to detect patterns and preferred channels for consumption. It can use historical and real-time data to determine that I, a frequent business traveler and coffee addict, may welcome a real-time message that my favorite coffee shop is around the corner. My dad would not welcome this interaction. He brews his coffee at home and will respond to a coupon in the mail. Which can also include incentives for other items he might buy on his next grocery outing. The machine is optimizing activities for each customer across known channels (digital, paper, brick and mortar). It won’t, however, independently create a new interaction channel that doesn’t already exist.
In simple terms, machine learning is particularly suited to problems where:
• Applicable associations or rules might be intuited, but are not easily codified or described by simple logical rules
• Potential outputs or actions are defined but which action to take is dependent on diverse conditions which cannot be predicted or uniquely identified before an event happens.
• Accuracy is more important than interpretation or interpretability
• The data is problematic for traditional analytic techniques. Specifically, wide data (data sets with a large number of data points or attributes in every record compared to the number of records) and highly correlated data (data with similar or closely related values) can present problems for traditional analytic methods.
Subscribe To Our Newsletter
Machines can be programmed to learn by studying data to detect patterns and by applying known rules
A practiced machine learning algorithm could recognize the face of a known “person of interest” in a crowded airport scene, thereby preventing the person from boarding a flight—or worse. Social media platforms utilize machine learning to automatically tag people and identify common objects such as landmarks in photos. Why Is This a Machine Learning Problem? Image data is complicated and the number of pixels in each image makes the data set wider than it is deep. Pixels close to one another have similar values making the data highly correlated. Images of the same subject have multiple subtle (and not-so-subtle) variations. Of course, you can easily recognize people known to you – and those that aren’t – in pictures; even when they have different expressions, poses, or clothes. You can also identify “like” items both conceptually (i.e., animal, mineral, or vegetable) and concretely (i.e., dog, cat, fish). But can you translate that knowledge into simple steps and discrete rules for how you made the match?
As Dr. Steve Sherer pointed out in a recent webinar with our CEO Marc Lamoureux, Machine learning can help discover what genes are involved in specific disease pathways. Machine learning can be used to determine which treatments will be most effective for an individual patient based on their genetic makeup, demographic and psychographic characteristics.
Why Is This a Machine Learning Problem?
Genomic data is wide: every person has more than 20,000 genes. As a result, the number of genes (data points) in an individual record is always larger than the number of people (records) in any data set. A number of factors add to the complexity. Including, but not limited to: the high degree of variation within each of those 20,000+ genes. The fact that your relatives have similar genomes (making them highly correlated). That relatively few individuals may suffer from a given disease making the data pool extremely shallow. Last but not least, genes in isolation may not predict health outcomes or disease expression. Biochemical, environmental and other factors must also be considered, thereby requiring integrated data from multiple, diverse sources.
Machine learning can identify the best routes from point A to B, predict transit conditions and travel time and predict the best route based on current, evolving road conditions. Machine Learning can drive a car without requiring input from a driver. Why Is This a Machine Learning Problem? Driving is a complicated but well-bounded problem. There are, in fact, a limited number of actions a vehicle may take: start, stop, go forward, go backward, turn, speed up and slow down. However, the decision to take any action is influenced by numerous factors including but not limited to road conditions, weather conditions, presence and behavior of other vehicles, two-legged persons and their four-legged friends, and the rules of the road – just to name a few. While a human driver instinctually assesses all these inputs on the fly, capturing discrete rules for every possible combination is near impossible.